The Rise of AI in Energy Management: Part Two
- Written by John Dirkman, P.E.
- April 29, 2024
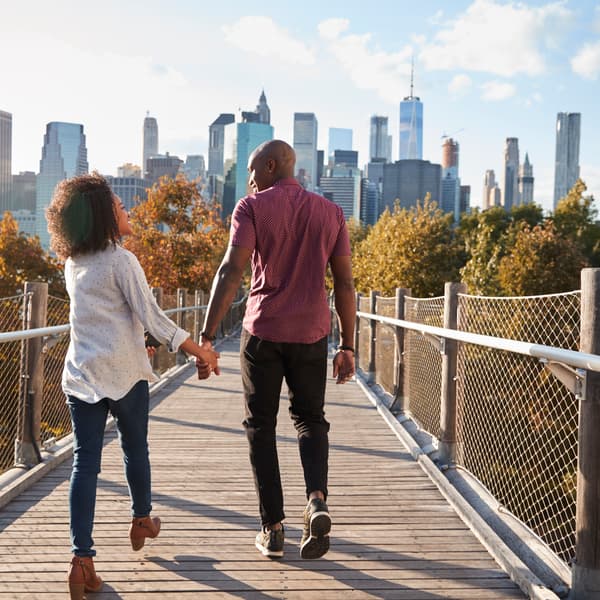
Imagine a world where energy management is more than a reactive response to fluctuations in demand and supply. One where every kilowatt of power is anticipated, every potential outage averted, and every renewable resource maximized to its fullest potential. Welcome to our smart grid solutions work at Resource Innovations, where cutting-edge AI applications are reshaping the landscape of energy management. Through our Grid360 platform for dynamic energy forecasting and its intricate optimization algorithms, Resource Innovations works closely with utilities and energy providers to transform how we power our world.
Resource Innovations’ AI Solutions
Grid360 and energy forecasting: Load and DERs (distributed energy resources) forecasting are used to generate load and generation predictions for a power system or its subsystems. Resource Innovations’ Grid360 platform offers short-term (hours to days) and long-term (months to years) load and DER forecasting. Utilizing historical data and weather parameters, it predicts load and generation at various system levels. Its AI-driven approach employs artificial neural networks for short-term forecasts and a flexible framework for long-term predictions. Integrated weather and sensor data enhance accuracy, crucial for demand response and grid stability planning and operations. Grid360's state estimation and power flow algorithms ensure reliable network operation, incorporating Grid360's forecasts for more precise calculations. With customizable profile creation and automatic load association, it empowers users to make informed decisions for efficient power system management.
Grid edge: Grid edge solutions utilize AI and advanced control algorithms to optimize real-time grid operations. By integrating DERs like solar panels, wind generation, battery storage systems, and electric vehicle charging stations, AI-based grid edge analytics help utilities balance supply and demand, enhance grid stability, and maximize renewable energy utilization. Through a Department of Energy grant project with Arizona State University, Resource Innovations employs AI in various applications, including:
- Determining optimal battery storage system placement, sizing, and charge/discharge times.
- Placing micro-PMUs in distribution feeders to estimate system state using deep neural network processing.
- Identifying ideal locations for solar panel smart inverters to improve voltage in different scenarios.
Grid data quality: AI enhances grid data quality, crucial for outage prediction, transformer loading, and voltage drop calculations. Through the same Department of Energy grant project with Arizona State University, Resource Innovations deploys AI by:
- Providing an integrated multi-stage spectrum clustering algorithm to model secondary distribution network topology accurately.
- Utilizing AMI data to validate meter-to-transformer connection and ensure precise secondary distribution network topology modeling.
- Incorporating county parcel data to validate and refine the geographic location of meters, further enhancing accuracy.
Grid resilience: Additionally, AI is used to improve the resilience and reliability of the grid. As part of a project with Arizona State University sponsored by the BIRD Foundation, AI is used for an advanced Temporal Graph Convolutional Network Multi-Agent Reinforcement Learning technique. This is a powerful deep learning architecture, capable of analyzing power grid topology and temporal dynamics. It creates a more compact representation of the grid that is better suited for the reinforcement learning agent analysis. In this project, we use AI to analyze a combination of grid attack vectors and mitigation actions:
- Topology actions: Changing the topology of substations
- Status actions: Power-line switching
- Re-dispatch actions: Changing the operating schedule of power plants
- Curtailment actions: Limiting the production of renewable generators
- Set-storage actions: Changing the role of storage units from loads to generators and vice versa
In a related project to detect and mitigate utility cyberattacks, Support Vector Regression is used to predict loads at the bus level and Support Vector Machines are used to detect and then mitigate cyberattacks to SCADA systems.
As we navigate the complexities of modern energy grids, one thing is clear: the future of energy management lies in the hands of those who innovate. From enhancing grid resilience to optimizing renewable energy utilization, the real-life examples of AI applications used at Resource Innovations illustrate the power of facilitating change.
If you want to continue the conversation, contact me at jdirkman@resource-innovations.com.
Read part one of this blog series here.
Related Energy Resources
Lead the charge to power change with Resource Innovations' smart grid insights.